The Laura P. and Leland K. Whittier
Virtual PICU
Creating a common information space for pediatric critical care
The VPICU Mission
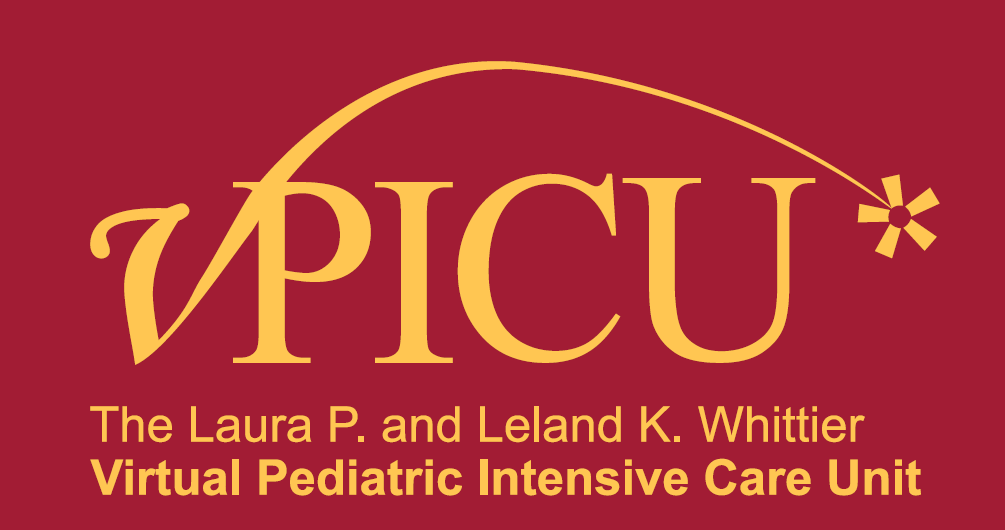
To create a common information space for pediatric critical care by:
- Developing & providing high quality pediatric ICU datasets
- Developing & applying advanced computational algorithms including AI/ML to extract meaningful and actionable information from data
- Collaborating with various clinicians at CHLA and other pediatric institutions
- Engaging with the pediatric critical care community through education, publications, and workshops